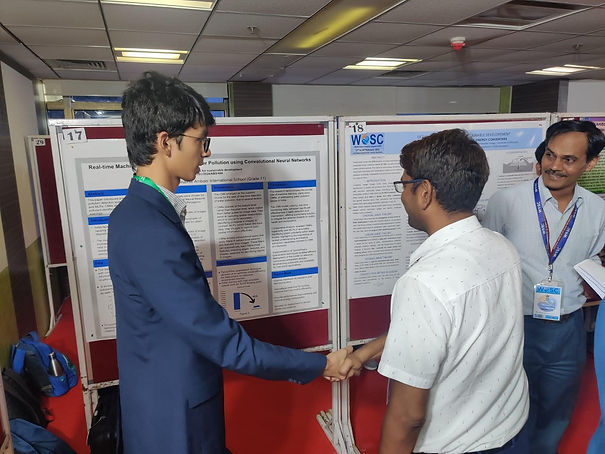
Research
Real-time Machine learning detection of water pollution using Convolutional Neural Networks.
​
Water pollution poses a significant threat to public health and ecosystems in India, necessitating effective early warning systems for timely intervention. My research study explores the utilization of machine learning techniques, specifically Convolutional Neural Networks (CNNs) and Multi-Layer Perceptrons (MLPs), for real-time detection of water pollution events. Leveraging real-time monitoring data, my ML model provides timely alerts to communities and authorities in local languages, enabling proactive measures to mitigate the impacts of pollution. I used a comprehensive dataset comprising 2000 images annotated with pollutant information. CNNs were trained to detect pollutants through bounding boxes which mapped the pollutants, achieving real-time inference capability in under 20 milliseconds.
By leveraging the model's ability to analyze real-time data and detect objects, timely alerts were generated. This research underscores the potential of machine learning in combating water pollution and fostering sustainable water resource management in the world. By empowering communities and authorities with timely and accurate information, this approach has the potential to play a crucial role in safeguarding public health by providing access to clean drinking water (SDG-6) and protecting vulnerable ecosystems. This system not only aids in predicting pollution levels but also facilitates timely interventions, benefiting the agricultural economy, mitigating disease spread, and reducing mortality rates.
Created a buoy to house my research algorithm on early warning systems to detect water pollution. Incorporated waterproof camera, smartphone attachment, to record images & detect surface pollutants.
​
​
​
​
​
​
​
​
Ocean Watch​​
​
Ocean Watch, is a platform that uses real time API to get geospatial data about the world’s ocean current’s to build a Monte Carlo Simulation algorithm, which finds the expected location of high concentrations of trash in the ocean. Then you can simulate the path of garbage particles at any given point on the map and it will track its location. Using Ocean Watch communities can track the most probable location where ocean pollutants will end up, leading to a more efficient and targeted cleanup by the governments
As there are many large ships in the ocean already cleaning up trash Ocean Watch will just help maximize their productivity.
The prediction algorithm in 'Ocean Watch' utilizes satellite data on ocean currents and speed to simulate the trajectory of pollutants in the ocean. Through data preprocessing and merging, the code calculates the u and v components of velocity vectors, representing east-west and north-south flow. The application visualizes this data with a quiver plot, displaying arrows that depict the direction and magnitude of currents at various locations. By simulating particle movement based on the calculated components, 'Ocean Watch' enables us to predict where pollutants might travel from any point in the vast ocean.
​
Research On Algal Blooms.
​​
XG-Boosting Model for the Detection of Algal Blooms using Sentinel 2-A Satellite Data.
This research paper addresses the pressing issue of algal bloom detection and mitigation by proposing an integrated approach utilizing machine learning and image processing techniques.
Using Python to develop an end to end workflow which could detect the severity of an algal bloom given the coordinates of any water body in real time using multispectral data from the Sentinel 2A satellite. Trained the model on 16000 data points and had an MSE of 1.216 while detecting the cyanobacteria density which is below the NASA's Tick Tick bloom benchmark.​​
By using convolutional neural networks (CNNs) for water body segmentation and developing predictive models for algal bloom identification, our study aims to enhance accuracy and efficiency in monitoring and managing water quality degradation.

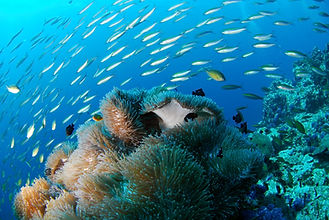